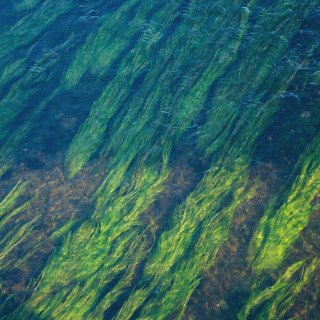
Hashtags like #covid19 and #coronavirus help us stay up to date on the developments of the new coronavirus pandemic. But beyond breaking news, tweets also offer a glimpse into the emotional side of the COVID-19 crisis. Are people feeling worried, sad, or confident about the virus? Xiangliang Zhang, an associate professor of computer science at King Abdullah University of Science and Technology (KAUST), wants to find out. Xiangliang runs the Machine Intelligence & kNowledge Engineering (MINE) group at KAUST where she builds computer models that identify Twitter users’ interests and track how they evolve. Now she’s turning her attention to COVID-19 and using machine learning to track the changing emotional response to the global crisis.
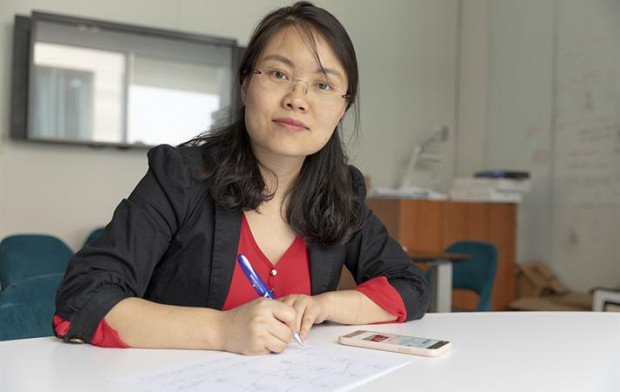
She and her team have collected millions of tweets from the last three months in English, Italian, French, Spanish, and Arabic and are using deep learning models to try and identify the emotions conveyed in the tweets. They built specialized models for each of the five languages and trained the models on the tweet data sets until the models could accurately identify how the author of the tweet felt about coronavirus. “We have very accurate models for English and Spanish, but our Arabic model isn’t very good. We’re now looking at how we can use the pre-trained English and Spanish models to help the Arabic model,” says Xiangliang. “This is an advanced topic in machine learning called transfer learning and also recently studied as meta learning.”
Transferring knowledge in different learning tasks is something the human brain does remarkably well, especially when it comes to language learning. We are able to use the knowledge we have of one language to recognize patterns in a second similar language. Xiangliang gives a personal example, “I learned French when I was doing my PhD and it was very difficult for me because I only know Chinese and English. There were some Italian and Spanish students in my class too and after a few weeks, they could already have basic conversations in French, but I was still struggling with single words.” Xiangliang and her students want their language models to act more like human brains. Not only will transfer learning make the models perform more accurately, it will also help the group develop models for less common languages where there are fewer tweets to use as training samples.
This kind of “thinking” is a step closer to artificial general intelligence (AGI), the hypothesized future of machine learning and AI systems. The “general” part of AGI means that the AI system can move beyond pattern recognition and make generalizations or analogies to solve a problem, just like a human brain would. “Given a single task, a single problem, we can always solve it if we have enough data and our model is good enough,” Xiangliang explains. “But the more challenging task is to make the current system generally intelligent enough to adapt to the new problem. People have already started working on this and I believe it will be the focus of the whole community in the next few years.”
Xiangliang has been at KAUST since 2010, a year after the university opened. But despite its age, the university had already recruited a group of renowned researchers to its faculty by the time Xiangliang applied. She was impressed. “Although KAUST was a very new university, people already had confidence about the future. That makes it a perfect place for a new PhD to start a new career,” she said. “I realized that if I worked at KAUST I would have the chance to collaborate with researchers who are at the top of their fields.”
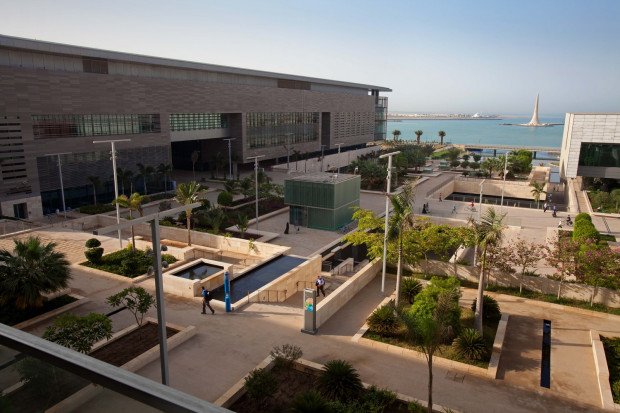
And she did just that. In her time at KAUST, Xiangliang has collaborated with some of the university’s big-name professors on several interesting machine learning application problems. She built machine learning models for a marine science group that used drone photos to monitor plastic litter along the coast of the Red Sea. She made another model that detects sea turtles in seawater. Xiangliang has also collaborated with geophysics researchers on seismic data and is currently working with Saudi Aramco to use machine learning to optimize oil production and detect anomalies in oil wells and oil fields.
Xiangliang enjoys being able to participate in diverse research projects and being part of such an international work environment. Over 110 nationalities are represented in the KAUST community—including the 1,100 students and 170 faculty members who all live and work on campus. Xiangliang believes that diversity of backgrounds, strengths, and expertise is necessary to enhance research. “It’s like what I was saying about AGI. We want our model to be adapted to environments, and the same goes for us, for our human brains,” she says. “We really want to have a diverse background and experiences because then our own intelligence can be diverse.”
Header image courtesy of KAUST
Continue reading